DOI: https://doi.org/10.59350/3pxva-3ev22
We have always envisioned QDR as more than a place to deposit (qualitative) data. QDR is also a resource for qualitative and multi-method social scientists working with data. As part of this vision, we regularly teach courses on data management. With varying line-ups, QDR experts have taught data management at the annual Institute for Qualitative and Multi-Method Research since 2013, where we also held individual data management consultations for the first time this year. We have taught workshops at IASSIST (2015, 2017), most recently together with our colleagues from UK Data; offered a webinar with more than 50 participants last year; and have given customized workshops by invitation from individual groups, such as the University of Kansas’s Work Group on Qualitative Research. Every time we teach such a course, we learn new things from the researchers we interact with – including better ways to teach data management. These are some of the lessons we learned.
Lesson 1: Sweat the Simple Stuff
The most frequent attendees of our workshops and seminars are graduate students. Graduate school includes learning about a lot of complex concepts and tools. It typically does not include simple tasks like “use a consistent rule for naming your files,” or “set up regular, automated, incremental backups,” and “have (at least) three copies in two locations.”
Lesson 2: Be Frank about Your Own Shortcomings
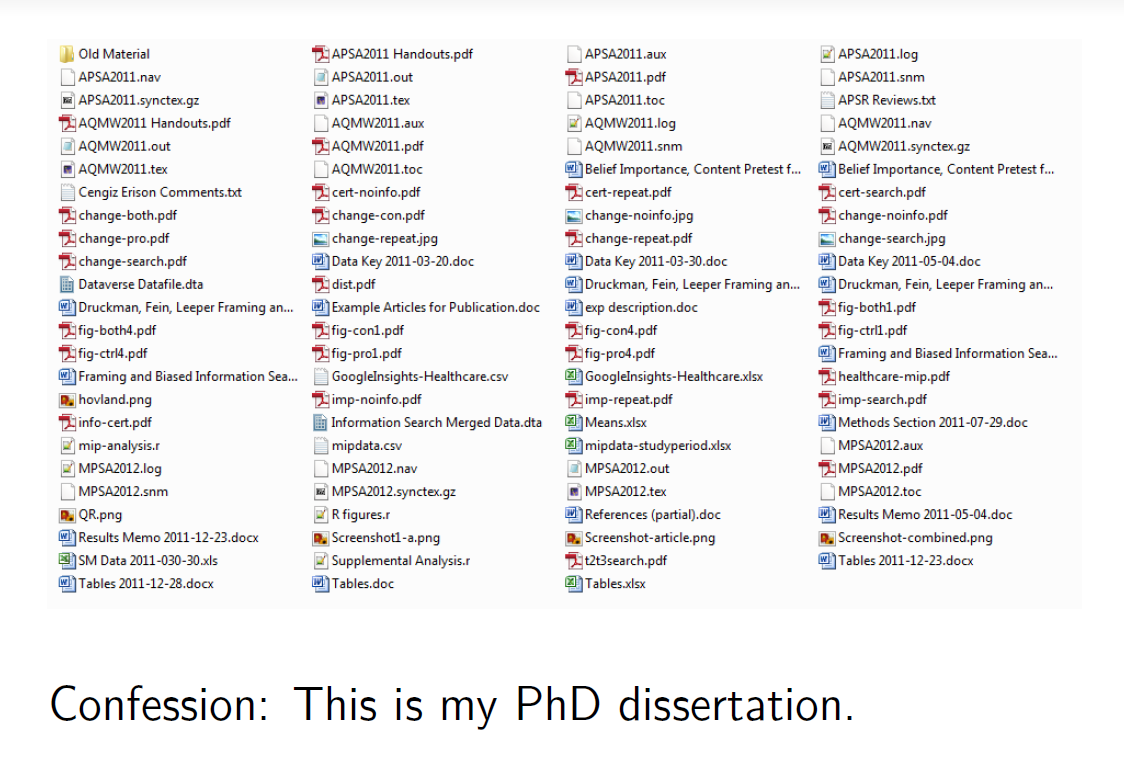
One of the most effective ways to impart a lesson is by showing your own “lessons learned.” Instead of claiming perfection in this and all past lives, highlight that you used to be terrible at this and learned the hard way. The above example is from a talk by Thomas Leeper, who is now a sought-after expert on transparent (quantitative) research.
Lesson 3: Make It Hands-on
This one is not going to surprise anyone: people learn best by applying tools and concepts. What are some of exercises to make data management “experiential”? One of our more recent discoveries is that having students assess data management plans (either samples or, ideally, those of peers) using a rubric is highly effective as a teaching tool. We have used the rubric developed by the Data Management Plans as A Research Tool (DART) for NSF data management plans with great success among qualitative researchers. In longer workshops, we had participants with developed research projects create a first draft of their data management plan using the DMP Tool, with which very few researchers are familiar.
We also use data prepared by UK Data to teach de-identification. Using (almost) real data highlights the challenges that the richness of qualitative data poses for de-identification. While we already make slides and select exercises available for teaching the management of qualitative data, we hope that we can add more of the data and materials we use for teaching for others to utilize.
Lesson 4: Cloud Watching
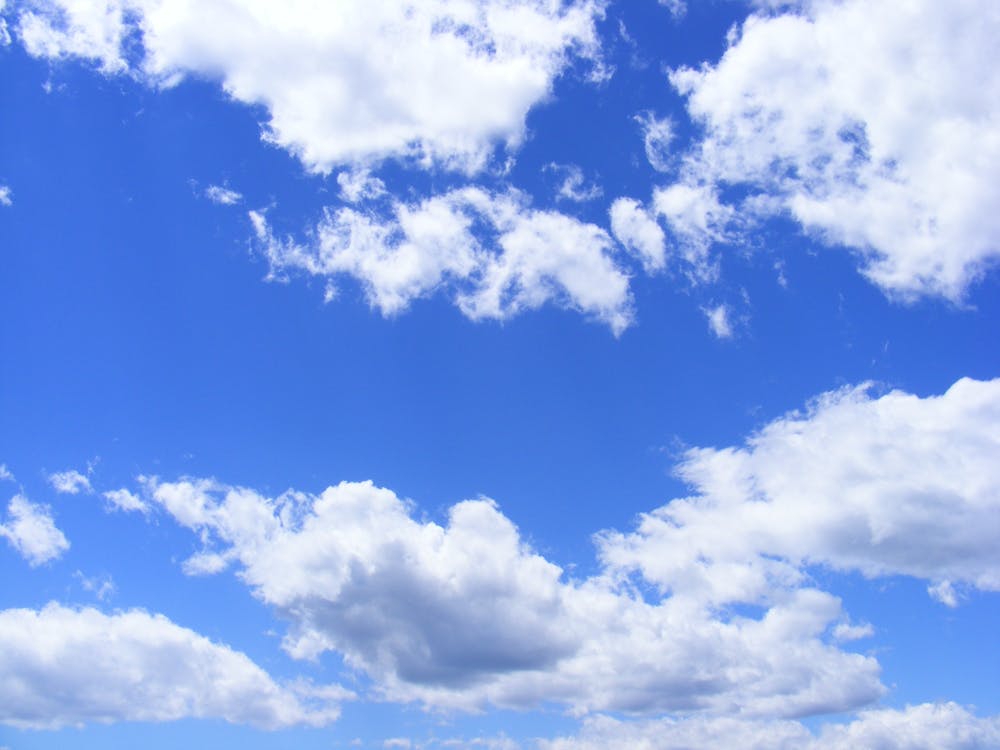
One of the questions we get most reliably in our courses concerns the use of cloud services such as Dropbox, Box.com, or Google Drive for storing research data during data collection and analysis. The right answer depends on a number of factors including legal aspects (e.g., storing personal data in the cloud is very problematic in the EU), the specific cloud service (professional / subscription-based products might offer greater control), and the nature of the research data (some data do not present concerns in this respect). Given these practical issues, librarians and repositories teaching data management need to be able to provide guidance on such services. At QDR, we now recommend cloud services in many cases because of the excellent backup functionality they provide combined with increasingly well-documented security and privacy infrastructures for established products, such as Dropbox Business.
Lesson 5: In the Long Term … Our Data Still Need Preservation
In helping qualitative researchers craft data management plans, we find that by far the biggest gaps are in sections about long-term storage and sharing. As a repository, we have an obvious interest in researchers sharing their data. But even without this, uhm, bias, the absence of clear planning for the moment a project is finished is worrying. For example, many researchers promise 10 years or more of data storage, without specifying a location where data will be stored, let alone any logistics that would assure preservation of the files or prevent file-format obsolescence.
Lesson 6: This Is Actually Useful!
Researchers often see the data management plans required by NSF and other funders as an annoying formality – yet another form to fill. But the (albeit self-selected) group of researchers we have taught ultimately find the data management plans to be quite useful. Many even develop genuine enthusiasm as they start feeling well equipped to write a data management plan and understand why they should do so in the first place. They start seeing the task as a way to help their own future selves.
Are you teaching DMP to researchers? What are some of the lessons you have learned? Tell us on twitter @qdrepository or by email.